Curriculum Analytics: Approaches to Structural Analysis
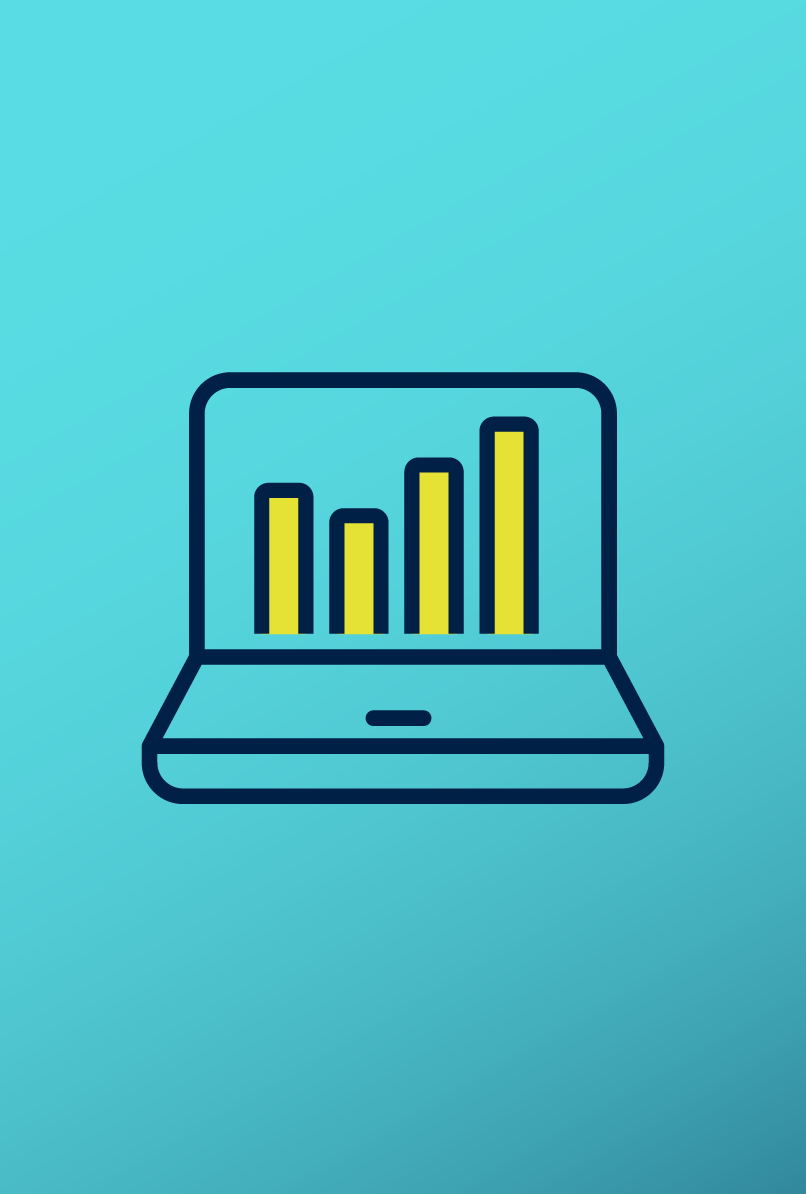
Institutional research (IR) offices are often tasked with providing information about student progression towards a degree. The nature of the institutional data available lends itself to providing significant insights into the structure of programs of study, student course-taking patterns, and outcomes at different stages of a curriculum. This information can be used to understand the impact a curriculum has on progression to a degree and to inform actions that can better support degree attainment (see De Silva et al., 2024, for a recent systematic literature review). Hence, Curriculum Analytics (CA), following the definition from De Silva et al., has become an important subfield of Learning Analytics “that aims to provide evidence-based decision-making on curriculum improvements (Hilliger et al., 2023), contributing to improving student learning, success in grades, student progress through the program, and ultimately, post-graduation job attainment (Li et al., 2023b).”
Considering the importance CA has in higher education, we provide here a description of four methodological approaches that focus on program structures and are based on institutional records regularly warehoused in data systems available to IR. Of course, CA can include analysis of course content, learning, and learning outcomes that are based on data not regularly available in the data warehouses of most institutions (for an in-depth discussion of theoretical frameworks underpinning structural approaches to CA, please see Fiorini et al., 2023).
All four approaches represent different applications of graph theory. Graph theory-based analytical frameworks lend themselves to representing and analyzing entities of a curriculum. These entities include degree-required courses and prerequisites as well as their relationships, such as whether a course is a pre- or corequisite of another course. The graphical representation of these relationships (in other words, the emerging network) can then be analyzed to reveal properties of the graph and highlight features that can affect the achievement of a successful transition to a degree or the identification of more efficient paths to a degree. The goal of an analysis of the curricular structure is to provide actionable insights to departments, faculty, staff, and students.
First, the Curriculum Prerequisite Network (CPN) can be used to highlight courses
Second, an effective approach to understanding the importance of course chains and identifying gatekeeping courses and their impact on student progression guides the analytical framework spearheaded by Heileman and colleagues. In this Degree Plans as DAGs approach, Heileman et al. (2018) and Wigdahl et al. (2014) extended the use of DAGs to analyze degree plans (Figure 1), developing metrics to quantify the rigidity of curricula and the constraints on student flow.
This approach is based on established rules that define how courses are interconnected in prerequisite chains. By modeling curricula in this way, researchers can identify structural attributes that impact student success. Heileman et al. (2018) liken this approach to explaining biological phenomena through their underlying chemistry and physics. Figure 1 shows a representation of a curriculum as well as an example of the metrics used to represent the complexity of individual courses within the curriculum, and the overall curriculum complexity as a sum of course complexity across all terms within a degree program.
The complexity metrics consist of a blocking factor (the number of courses later in the curriculum that cannot be taken until the initial course is passed); a delay factor (the longest pre- and corequisite chain of courses that the course is involved in); and a centrality factor (the number of prerequisite paths that directly enter into a course plus the number of courses it is a direct prerequisite for). If the course has no pre- or corequisites, its centrality factor is zero. The course complexity score is the sum of the blocking and delay factors for a course (Heileman et. al., 2017). Research has demonstrated that greater structural complexity in the curriculum is related to lower student GPAs and retention (Slim et. al., 2016).
Figure 1: An example DAG showing an Electrical Engineering degree plan.
Third, taking inspiration from Social Network Analysis, Dawson and Hubball (2014) applied this technique to student enrollments to uncover dominant curricular structures and pathways. In this case, the relationships between courses represent students’ enrollments, that is, two courses are connected if a student enrolled in both courses. This approach was used effectively to guide analytics in response to the Covid-19 emergency on the Bloomington campus at Indiana University (Deom et al., 2021). In Dawson and Hubball (2014), the method finds its power when paired with interactive tools to visualize data, which can be customized to show demographic and other characteristics of students and programs. By revealing these underlying structures, this approach can inform questions related to support provided to students of different demographics and quality assurance processes, as well as connect curricular paths to post-graduation success. This method highlights the potential of using student enrollment data to improve educational outcomes.
Fourth and finally, the Process Mining methodology (see Salazar-Fernandez et al., 2021) uses process maps and traces of student interactions with course requirements to represent the lived experiences of students as they interact with the requirements of their aspirational academic path. This approach, as discussed by Trčka and Pechenizkiy (2009), Fiorini et al. (2023), and others, interprets these interactions as part of a socio-educational environment shaping the student and their experience. Process mapping as an approach to CA is highly valuable, as it generates traces or characterizations of how students flow through the curriculum as a sequence of course enrollments (see Fiorini et al. 2023) that can repeat, thus removing the constraint related to the DAG representation of the network. This approach also allows for the representation of outcomes of a curriculum as a node in the network, providing an analytical connection between courses, enrollments and the flow of students to an end state like a degree in a specific major, in another major, or attrition (Figure 2). These process maps can be reviewed by faculty and administrators to understand the reality of how students interact with the curriculum and how it compares with what is expected given the theoretical setup of the curriculum course requirements. This methodology emphasizes the importance of understanding the curriculum as a network of interconnected events, rather than just a set of courses and prerequisites.
Figure 2: Example process maps showing student pathways through the introductory chemistry sequence at Institution A (top) and Institution B (bottom). Courses are Preparatory General Chemistry (PGC), General Chemistry (GC), Organic Chemistry I (OCI), and Organic Chemistry II OCII). The edges represent the percentage of enrollments from one course to another and transitions from a course to a final status that could be a Biology degree (Biology D), a STEM degree (STEM D), another degree (Other D), or that a student left (Left-Enrl), revealing areas of outflow from the curriculum path. Reproduced from Fiorini et al. 2023 (https://doi.org/10.3389/feduc.2023.1176876).
Building on graph-based analytics, IR professionals can derive further actionable insights for CA by supplementing process mapping, or other CA approaches, with information from machine learning algorithms. The process maps generate the complexity of how students interact with the curriculum, and institutional researchers can take that complexity and focus it to characterize the target outcome of the curriculum (i.e., retention in a specific major or discipline). This process allows for statistical and machine learning model building that enriches the analysis with new factors that explain the outcome by applying interpretable machine learning methodologies (Gopinath, 2021; Murphy, 2022; SHAPforxboost, 2023). The results can help identify the most important elements (e.g., grades in specific subjects or student background characteristics) that explain students’ progression in the curriculum and highlight priority areas for program development or curriculum changes.
Each of these methodologies provides a useful set of graphic tools to help faculty and administrators visualize and identify where the curriculum is creating unexpected roadblocks to student progression. However, it is advantageous to combine the tools with a structure that allows faculty to examine, test, and refine the curriculum to improve upon it. The Integrative Learning Design (ILD) Framework is one such useful tool for making these representations of curricular structures actionable. Hilliger et al. (2020) proposed an approach to CA based on the ILD framework, which involves continuous improvement and evaluation phases and views CA as a system involving stakeholders, technology, and knowledge.
By overcoming the limitations of graphically based characterizations, this approach emphasizes the importance of understanding curricula as networks of relationships. Faculty, administrators, and students are all involved in producing and evaluating actionable knowledge, making the curriculum a dynamic and evolving system. Another example employed by the Gardner Institute combines the Degree Plan as DAGs tool with a multi-institutional community of practice utilizing improvement-based techniques to allow faculty and staff to examine their curriculum, compare and discuss challenges and improvements with other institutions, and test and refine solutions to help improve student progression through the curriculum (Koch et. al. 2024). Each institutional team builds an evidence-based plan of action that focuses on student learning outcomes, removing barriers to degree completion. The institutions then work to implement and refine the plans of action over the course of a yearlong community of practice.
As institutions continue to work toward their strategic goals, IR professionals need an analytical toolkit to provide data about students’ pathways to degree attainment and identify strategies for removing barriers to completion. The methodologies described herein include graph-theory/social network analysis to define and quantify curriculum complexity, process mapping to generate traces of students’ flow through and out of a curriculum, and interpretable machine learning to help explain factors that influence progression to degree. In the spirit of AIR’s motto of IR “working at the intersection of people and data,” these approaches are most effective when done in collaboration with administrators, faculty, students, and other key campus constituents as represented in the approaches by Hilliger and her colleagues (2020) and the Gardner Institute (2024). Institutional research plays a crucial role in this space by bringing stakeholders together to use data-informed strategies for evaluating and improving curricula.
Bibliography:
Aldrich, P. R. (2015). The curriculum prerequisite network: Modeling the curriculum as a complex system. Biochem. Mol. Biol. Educ. 43, 168–180. doi: 10.1002/bmb.20861.
Dawson, S., and Hubball, H. (2014). Curriculum analytics: application of social network analysis for improving strategic curriculum decision-making in a research-intensive university. Teach Learn Inq 2, 59–74. doi: 10.2979/teachlearninqu.2.2.59.
Deom G., Fiorini S., McConahay M., Shepard L., Teague J. (2021) Data-Driven Decisions: Using Network Analysis to Guide Campus Course Offering Plans. College & University, Vol. 96, No. 4, pp. 2 - 13.
De Silva, L.M.H., Rodríguez-Triana, M.J., Chounta, I.A. et al. (2024) Curriculum analytics in higher education institutions: A systematic literature review. J Comput High Educ. https://doi.org/10.1007/s12528-024-09410-8.
Fiorini, S., Tarchinski, N., Pearson, M., Valdivia Medinaceli, M., Matz, R.L., Lucien, J., Lee, H.R., Koester, B., Denaro, K., Caporale N. and Byrd, W.C. (2023) Major curricula as structures for disciplinary acculturation that contribute to student minoritization. Front. Educ. 8:1176876. doi:10.3389/feduc.2023.1176876.
Gopinath, D. (2021). The Shapley Value for ML Models. What is a Shapley value, and why is it crucial to so many explainability techniques? Towards Data Science. https://towardsdatascience.com/the-shapley-value-for-ml-models-f1100bff78d1.
Heileman, G.L., Abdallah, C.T., Slim, A., and Hickman, M. (2018). Curricular analytics: a framework for quantifying the impact of curricular reforms and pedagogical innovations. arXiv preprint. arXiv:1811.09676.
Heileman G. L., Slim, A., Hickman, M. & Abdallah, C.T. (2017) Characterizing the complexity of curricular patterns in engineering programs. In ASEE Annu. Conf. Expo. Conf Proc., Vol. 2017-June. American Society for Engineering Education. https://peer.asee.org/characterizing-the-complexity-of-curricular-patterns-in-engineering-programs.
Hilliger, I., Miranda, C., Celis, S., & Perez-Sanagustin, M. (2023). Curriculum analytics adoption in higher education: a multiple case study engaging stakeholders in different phases of design. British Journal of Educational Technology. https://doi.org/10.1111/bjet.13374.
Hilliger, I., Aguirre, C., Miranda, C., Celis, S., and Pérez-Sanagustín, M. (2020). Design of a curriculum analytics tool to support continuous improvement processes in higher education. In Proceedings of the Tenth International Conference on Learning Analytics & Knowledge LAK’20. (New York, NY, USA: Association for Computing Machinery), 181–186.
Koch, A. K., Foote, S. M., Smith, B., Felten P., & McGowan, S (2024). Shaping what shapes us: Lessons learned from and possibilities for departmental efforts to redesign history courses and curricula. Journal of American History, 110 (4), 739-745. https://doi.org/10.1093/jahist/jaad356.
Li, X. V., Rosson, M. B., & Hellar, B. (2023b). A synthetic literature review on analytics to support curriculum improvement in higher education. EDULEARN23 Proceedings, 2130–2143. https://doi.org/10.21125/edulearn.2023.0640.
Murphy, A. Shapley Values – A Gentle Introduction. (2022). H20.ai Blog. https://h2o.ai/blog/shapley-values-a-gentle-introduction/.
Package SHAPforxgboost. (2023). https://cran.rproject.org/web/packages/SHAPforxgboost/SHAPforxgboost.pdf.
Salazar-Fernandez, J.P., Sepúlveda, M., Munoz-Gama, J., and Nussbaum, M. (2021). Curricular analytics to characterize educational trajectories in high-failure rate courses that lead to late dropout. Appl. Sci. 11:1436. doi: 10.3390/app11041436.
Slim, A. Heileman, G.L., Wisam, A., & Abdallah, C.T. (2016) The impact of course enrollment sequences on student success. Proc. – Int. Conf. Adv. Inf. Netw. Appl. AINA 2016-May (May 2016), 59-65. https://doi.org/10.1109/AINA.2016.140.
Trčka, N., and Pechenizkiy, M. (2009). From local patterns to global models: towards domain driven educational process mining. In 2009 Ninth International Conference on Intelligent Systems Design and Applications, 1114–1119.
Wigdahl, J., Heileman, G., Slim, A., and Abdallah, C. (2014). Curricular efficiency: What role does it play in student success? ASEE Annual Conference and Exposition, Conference Proceedings. doi: 10.18260/1-2—20235.
Stefano Fiorini Ph.D. Ph.D. is a Social and Cultural Anthropologist with the Research and Analytics team, a subunit within Indiana University’s Institutional Analytics office. He has extensive applied research experience in the areas
of institutional research and learning analytics. He has published in peer reviewed journals and conference proceedings and presented at national and international conferences (e.g. AIR Annual Forum, CSRDE, LAK), earning best paper awards from INAIR,
AIR and SoLAR.
Gina Deom has nearly 10 years of experience working in higher education data and research. She currently serves as a data scientist with the Research and Analytics team, a subunit within Indiana University’s Institutional Analytics
office. Gina has given several presentations at national and international conferences, including the SHEEO Higher Education Policy Conference, the NCES STATS-DC Data Conference, the Learning Analytics and Knowledge (LAK) Conference, and the AIR Forum.
Gina has earned a best paper award from INAIR, AIR, and LAK.
Dr. Brent Drake is the Senior Vice President for Operations and Research at the John N. Gardner Institute for Excellence in Undergraduate Education, a non-profit that partners with higher education institutions to improve student success. Prior to joining
the Garnder Institute he served for five years as the Vice Provost of Decision Support at the University of Nevada, Las Vegas, and before that he was with Purdue University for 17 years, spending the last five years of his tenure as the Chief Data
Officer. Brent also is the current chair of the Board of Directors of the Association of Institutional Research. He presents and publishes on a number of topics in higher education including motivational models related to student success, retention
enhancing programs, business intelligence and data analytics, enrollment and student completion predictive modeling, recruitment, enrollment trends and student success efforts.
Dr. Brandon Smith serves as an Associate Vice President with the Gardner Institute. He started his career as a member of the faculty at Midwestern State University and continued to Brevard College to lead their theatre program. While at Brevard,
Brandon also served as associate dean for student success. He joined the Gardner Institute on a full-time basis in 2021. He continues to teach, write, direct, consult on storytelling, and conduct research in both the arts and postsecondary systems
linked to student success. As a practitioner-researcher, Dr. Smith is particularly interested in utilizing improvement science tools to focus and catalyze organizational transformation. He authored a chapter in The Educational Leader's Guide
to Improvement Science: Data, Design, and Cases for Reflection, published by Stylus.